Next: 4. Memory bandwidth and
Up: 3. Some results
Previous: 3.1 daxpy and ddot
dgemm is a level 3 operation which performs a matrix
matrix product
where A, B, C are respectively
m x k, k x n and m x n matrices. The ASCI Red
implementation and ATLAS use a block method to perform matrix matrix multiply
and achieve good performances As we
see in figure
end figure
performances and acceleration are very good and remain as the size of matrices
increase.
Performances for dgemm are important because it's a building block for block LU
factorization. We know outline a block LU factorization method, the reader may refer
to [5] for an in-deep analysis and algorithms, and discuss how
multi-threaded version can be realized.
Figure:
dgemm performances with 1 and 2 processors
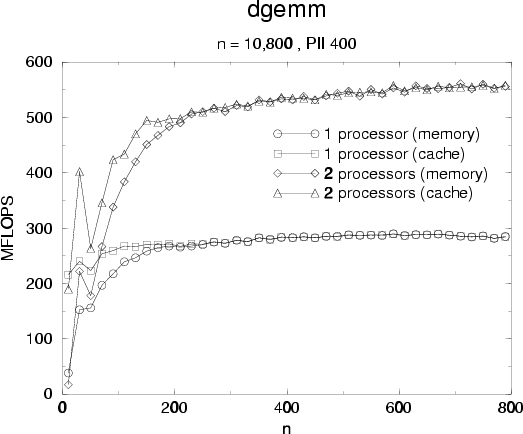 |
Figure:
dgemm acceleration for 2 processors
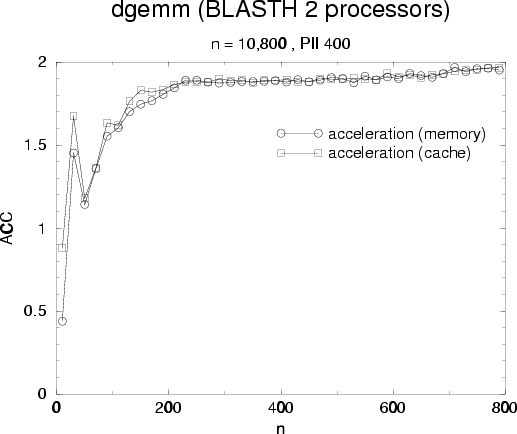 |
LU factorization is used to solve linear system like A.X=B by factorize
A into L.U where L is a lower triangular unit matrix and U is an upper
triangular matrix. Efficient LU methods rely on matrix matrix product and we
outline such method in the following.
For simplicity we suppose that the block size nb divide n which is the
size of the square matrix A and set N = n/nb. Each block of A is
numbered
.
The block LU performs as follow:
- 1.
- set k=1,
- 2.
- compute Ak,k=L.U and overwrite Ak,k with L and U using a non blocked LU
factorization,
- 3.
- apply row interchange in Ak,k+1:N and in Ak,1:k-1
- 4.
- solve
L.X=Ak,k+1:N and overwrite Ak,k+1:N with the solution,
- 5.
- solve
X.U=Ak+1:N,k and overwrite Ak+1:N,k with the solution,
- 6.
- update
Ak+1:N,k+1:N with
Ak+1:N,k+1:N = Ak+1:N,k+1:N -
Ak+1:N,k.Ak,k+1:N,
- 7.
- if k<N set k=k+1 and go to step 2.
Step 2 uses subroutine dgetf2 from LAPACK, row interchange is done
using dlaswp, step 3 and 4 use dtrsm (triangular solve with multiple right hand
side) and step 5 uses dgemm. It is important to note that most computation is
done in steps 4 and 5 which use level 3 BLAS and represent 1-1/N2 of the
floating point operations issued (see[5]). Figure
presents task dependencies in block LU for step k, it outlines a
graph dependency for a parallel implementation. At this point we have two
choices for multi-threaded version:
- We can use a dependency graph of task with each processors searching for
ready tasks (tasks for which each ancestor is done) and executing them. By
splitting the solve and matrix multiply tasks into smaller ones we can see
that LU for step k can be done while matrix multiply for step k-1 is
finishing since
Ak,k = Ak,k - Ak,k-1.Ak-1,k is done at step k-1.
- We can also perform the LU task on one processor and then use
multi-threaded version of dlaswp, dtrsm and dgemm.
Figure:
LU factorization scheme for the step k
|
The first solution requires to construct the dependency graph and more complex
synchronization scheme but always based on synchronization variable. Construct
the graph and searching for ready task may be a serious overhead thus limiting
acceleration. The second solution let the LU task be a sequential bottleneck
and use parallelism only on level 3 operations.
For implementation we choose the second solution because it's simple and LU
bottleneck is in fact very small since it represents only 1/N2 of the
overall job. Results for our block LU factorization are presented
figure
and figure
: we use dgetrf from
ATLAS as a reference code for our sequential block LU. Acceleration figure
presents two curves; one presents acceleration obtained by the multi-threaded
block LU, the other (ideal acceleration) is computed using the sequential code
by looking for time spent in dgetf2 and in level 3 operation:
For
acceleration is relatively closed to ideal acceleration but
smaller case acceleration is far from ideal due to synchronization cost.
Figure:
block LU performances with 1 and 2 processors
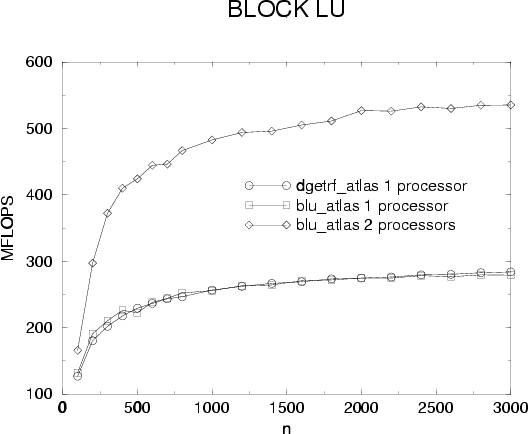 |
Figure:
block LU acceleration with 1 and 2 processors
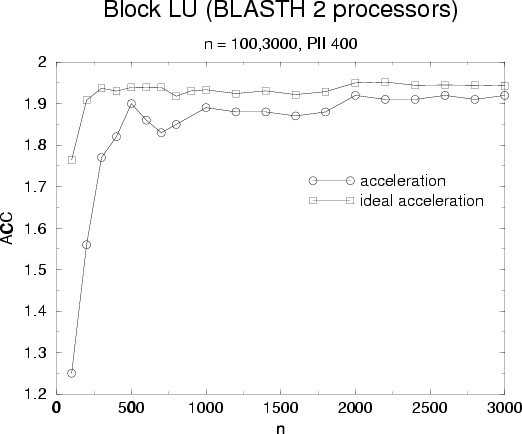 |
Next: 4. Memory bandwidth and
Up: 3. Some results
Previous: 3.1 daxpy and ddot
Thomas Guignon
2000-08-24