2nd USENIX Symposium on Internet Technologies & Systems, 1999   
[Technical Program]
Pp. 1324 of the Proceedings |  |
Hierarchical Cache Consistency in a WAN1
Hierarchical Cache Consistency in a WAN1
Jian Yin, Lorenzo Alvisi, Mike Dahlin, Calvin Lin
Department of Computer Sciences
University of Texas at Austin
yin,lorenzo,dahlin,lin@cs.utexas.edu
This paper explores ways to provide improved consistency for
Internet applications that scale to millions of clients. We make four
contributions. First, we identify how workloads affect the scalability
of cache consistency algorithms. Second, we define two primitive
mechanisms, split and join, for growing and shrinking consistency
hierarchies, and we present a simple mechanism for implementing them. Third,
we describe and evaluate policies for using split and join to address
the fault tolerance and performance challenges of consistency hierarchies.
Fourth, using synthetic workload and trace-based simulation, we
compare various algorithms for maintaining strong consistency in a
range of hierarchy configurations. Our results indicate that a
promising configuration for providing strong consistency in a WAN is a
two-level consistency hierarchy where servers and proxies work to
maintain consistency for data cached at clients. Specifically, by
adapting to clients' access patterns, two-level hierarchies reduce the
read latency for demanding workloads without introducing excessive
overhead for
nondemanding workloads. Also, they can improve scalability by orders
of magnitude. Furthermore, this configuration is easy to deploy by
augmenting proxies, and it allows invalidation messages to traverse
firewalls.
To improve performance and reduce bandwidth, caching has become a
ubiquitous Internet technology. However, web caching introduces the
problem of maintaining consistency. With weak notions of consistency
users can observe confusing data, and innovative web services--such
as agents, robots, and distributed databases--will likely produce
incorrect results. Furthermore, consistency polling can increase
server load, increase latency, and reduce the effectiveness of
large-scale caches [9,5]. Thus, improved consistency
will become increasingly desirable.
Callback-based consistency
can be used either to provide strong consistency--which guarantees
that a client's read of an object returns the latest completed write
of that object--or best effort consistency--which attempts to
invalidate stale data when it changes and which can limit the amount
of time during which a client unknowingly accesses stale
data [20]. However, simple callbacks are unacceptable for a
WAN because servers may be forced to delay their writes
indefinitely when there are client
failures or network partitions.
In previous work, we have shown how
to combine strong consistency with timely server writes by using Volume Leases [20], a generalization of the original notion
of leases [7].
By coordinating per-volume and per-object leases, the Volume Leases
algorithm presents a fundamental abstraction that provides a mechanism
for enforcing strong consistency semantics that is separate from the policy
question of when to renew volume leases. We focus on a
policy where clients fetch volume lease renewals on demand when they
access a volume; in the future we plan to explore other policies such as
prefetching or multicasting lease renewals.
Our earlier study uses trace-driven
simulations and shows that Volume Leases performs well compared to
object leases and polling.
The key questions that this paper answers are (1) how large a system
can Volume Leases accommodate, and (2) what techniques can be used to
scale to even larger systems? To answer these questions, this paper
explores ways to accommodate popular web servers with millions of
clients by combining Volume Leases with hierarchies.
Adding hierarchy to callback-driven consistency can yield
three benefits. First, latency can be reduced if clients can register
callbacks or renew leases by going to a nearby node in the consistency
hierarchy rather than to the server. Second, hierarchy can improve
network efficiency by forming a multicast tree for sending
invalidation messages to caches and a reduction tree for gathering
their replies. Third, hierarchy improves server scalability by
distributing load and callback state across a collection of nodes.
However, using hierarchy for scalable consistency introduces its own
challenges. Availability may suffer because hierarchical structures
consist of multiple nodes that can fail independently. Also,
latency can increase if the hierarchy must be traversed to satisfy requests.
Finally, it is unclear how best to configure the hierarchy.
This paper develops solutions for hierarchical consistency and
addresses these issues. We make four
contributions. First, we identify and quantify the ways in which
specific characteristics of data-access workloads affect the
scalability of cache consistency algorithms. Second, we define two
primitive mechanisms, split and join, for growing and
shrinking hierarchies, and we show how these primitives can be
implemented with a simple mechanism already present in the Volume
Leases algorithms. Third, we describe and evaluate policies for using
split and join to address the fault tolerance and performance
challenges of hierarchies. Fourth, we examine and compare
algorithms for maintaining consistency in a range of hierarchy
configurations.
We explore three configurations. First, in generic
hierarchy, consistency proxies can be placed anywhere in the
Internet. The second configuration, server-proxy-client, is designed to
exploit widely deployed web proxies, which serve as gateways between
enterprise LAN and web servers to improve security and network
efficiency. In this configuration, web proxies are augmented to serve
as consistency proxies that forward invalidation messages from
web servers. This addresses the engineering challenge introduced by
firewalls that generally prevent external machines from sending
invalidation messages directly to clients within the firewall. The
third configuration is the server cluster configuration in which
hierarchy is introduced only within a LAN cluster of servers to
improve scalability.
We evaluate our algorithms using simulation. To study scalability and
to evaluate how the system is affected by different workload characteristics,
we first use a series of synthetic workloads. To calibrate these
results with realistic workloads, we also examine some smaller
trace-based workloads. Overall, we find that even without
hierarchies, Volume Leases can scale to services with tens of
thousands of clients; with hierarchies, scalability beyond millions of
clients appears feasible.
The thesis of this paper is not that all servers should provide strong
consistency, but rather that for Internet-scale systems, strong
consistency is feasible for a wide range of applications. We envision
flexible systems where either servers or clients can specify the
consistency semantics for their data [10].
The algorithms described here can also be used to
provide best effort consistency where servers make a best effort
to notify clients of changes to cached data, but servers do not delay
writes. We discuss best effort versions of Volume Lease consistency
algorithms in detail elsewhere [20].
The rest of this paper proceeds as follows.
Section 2 discusses a few ideas that are needed to
understand this work. Section 3 describes our new
algorithm whose performance we evaluate in Section 4. We
close by discussing related work and drawing conclusions.
Background
This section describes four concepts necessary to understand hierarchical
consistency: callbacks, leases, the Volume Leases algorithm, and reconnection
under Volume Leases.
In server-driven consistency, a client registers callbacks with
a server for objects that it caches [9,15]. Before
modifying an object, a server sends invalidation messages to all
clients that have registered interest in that object. The advantage
of this approach is that servers have enough information to know
exactly when cache objects must be invalidated. By contrast, in
client-driven consistency schemes, such as those currently used in NFS
and HTTP, clients periodically ask the server if objects have been
modified. This creates a dilemma for the client. A short polling
period increases both server load and client latency, while a long
polling period increases the risk of reading stale cache data.
However, there are two challenges for server-driven consistency in
large distributed systems. First, scalability is an issue, because
large numbers of clients lead to large server state and large bursts
of load when popular objects are modified. Second, performance in the
face of failures is an issue because servers cannot modify an object
until all clients have been notified that their cached copies are no
longer valid. Because of this requirement, server writes can be
delayed indefinitely while the server waits for acknowledgments from
unreachable clients.
These challenges can be addressed by introducing leases [7]. When a client registers a lease with a server, the
lease specifies some time T during which the server will notify clients of
updates. Leases improve scalability because servers need to
track only active clients, and they improve fault tolerance because even if a
client is unreachable, writes are delayed only until the client's
lease expires. The lease length T presents a trade-off. Long
leases minimize the overhead of renewing leases, while short leases
reduce server state and improve failure-mode write performance.
Leases do not perform well for web workloads because the interval
between a client's reads is typically long, so object leases must be
long to amortize the cost of lease renewals across multiple reads [20].
The Volume Leases algorithm introduces the notion of a volume, which
is a collection of objects that reside on the same server. The algorithm
associates a lease with each volume as well as with each object. A
client's cached object is valid only if both its object lease and
corresponding volume lease are valid. The Volume Leases algorithm
uses a combination of long object leases and short volume leases to
resolve the tradeoff with lease lengths. Short volume leases allow
servers to write quickly in the face of client and network failures:
since clients can't read an object when its corresponding volume lease
is invalid, in the worst case the server waits only for the the short
volume lease to expire before modifying an object. While the cost of
renewing short volume leases is amortized across the number of objects
that reside in the same volume, long object leases minimize the
overhead of renewing object leases.
In the Volume Leases algorithm, a server maintains a list of
unreachable clients whose volume leases expired while the server was
attempting to invalidate an object lease. We call this list the unreachable list. After an unreachable client recovers or its network
connection to the server is restored, the next time that client
tries to renew its volume lease the algorithm uses a reconnection
protocol to restore consistency between the client's and server's
lists of current object leases.
Because the reconnection protocol is a key building block for
hierarchical caching, we describe it in detail. Each server maintains
an epoch number. Whenever a server recovers from a crash, it
increments the epoch number and logs that number to stable storage
before proceeding with normal operations. All messages from a server
to its clients include the epoch number. When a client receives a
message from a server, it records the server's epoch number. When
a client sends a volume lease request to a server, it always includes
the last known epoch number for that server.
A server grants a volume lease only if the epoch
number in the request matches its current epoch and if it has not
marked client unreachable. If the client's epoch
number does not match or if the client is marked unreachable, the
server sends the client a reconnect request. In response to such a
request, a client sends the server the list of objects it currently
caches and the version numbers of these objects. The version
number associated with an object is an integer that the server
increments whenever it modifies the object. The server then compares
the version numbers of the cached objects and server's objects and
grants object leases to all objects whose versions match. The server
invalidates all other cached objects then grants the volume lease.
All these tasks can be accomplished with one message from
the server to the clients. When the client finishes updating its
object leases, it sends a connect message back to the server, which
then removes the client from the unreachable list.
Algorithms
We first describe a naive algorithm based on a static
consistency hierarchy and discuss its performance and fault tolerance
properties. Next, we present two primitive mechanisms, split and
join, for reconfiguring the hierarchy. These mechanisms can be
constructed with trivial additions to the basic Volume Leases
algorithm. We then describe policies that use these mechanisms to
enhance the fault tolerance and performance of the basic static
hierarchy.
Both the static and dynamic versions of the algorithm assume that
nodes participating in the consistency service have been identified
and organized into an initial hierarchy. This study does not specify
a particular mechanism for doing so. For some systems, constructing
the hierarchy manually suffices; for some, such as the
server-proxy-client configuration in
Section 4.3, automatic construction is trivial; for
others, more sophisticated automatic strategies such as those
described by Plaxton et. al [16] might be required. This
hierarchy may be embedded on current clients and proxies, it might be
coincident with a larger cache hierarchy [2] or it
might be part of a separate data-location-metadata
hierarchy [6,18].
Our consistency hierarchy is a tree structure of interconnected nodes.
We refer to the root as the origin server, to the leaves as clients, and to the intermediate nodes as consistency servers.
Each node runs the standard Volume Leases algorithm; each
intermediate node acts both as a client and as a server, treating its
parent as its server and its children as its clients. Each node thus
satisfies lease requests from its children by returning a valid lease
if it has one cached, or--if it does not--by requesting a lease from
its parent, caching the lease, and returning the lease to its child.
Similarly, each node passes to any children that have valid leases the
invalidation messages that it receives.
Such hierarchies have the potential to improve performance by reducing
both server load and the latency of client lease renewals. In the
Internet, a popular site might serve millions of clients, and by using
a hierarchy, a server tracks and communicates with only its immediate
children. This reduces memory state, average load for lease renewals,
and bursts of load when popular objects are modified. In essence,
the consistency hierarchy forms a multicast tree for sending
invalidation message and forms a reduction tree for gathering
replies. By the same token, if clients can renew leases by going to
nearby intermediate consistency servers rather than to the root
server, read latency and network load may be reduced.
However, the use of leases in the hierarchy is not guaranteed to reduce
either server load or latency. When volumes are popular and frequently
accessed, it is likely that consistency servers will hold valid leases and
will respond to client requests without consulting their parents, and it is
likely that the hierarchical ``multicast'' will achieve a large fan-out and
significantly reduce server load. However, for unpopular or infrequently
accessed volumes, the time between accesses to consistency nodes is likely to
be longer than the volume lease, so the cached leases may often have expired
when they are accessed. In these cases, many messages would traverse
the entire hierarchy, increasing the average read latency without reducing
server load.
A second problem with a static hierarchy is reliability. The hierarchy
consists of a large number of nodes that can fail independently, and one node
failure can effectively disconnect a subtree.
The solution to both problems is to reconfigure the consistency
hierarchy dynamically without breaking consistency guarantees. We
propose a mechanism that uses two primitives: join, which removes an intermediate node from the hierarchy, and split, which adds an intermediate node to the hierarchy. Both
primitives work on a per-volume basis--in our system different
volumes can use different hierarchies.
Join and split can be trivially implemented using mechanisms already
required by the Volume Leases algorithm. Recall that join removes a
node from the hierarchy, connecting the children of the node directly
to the node's parent. To implement join we augment the volume epoch
number to include the parent node's identity. When a child
initiates a join for a particular volume, it simply begins using its
former grandparent as a parent. The volume epoch number held by the
child will not match its new parent, so the new parent initiates
the standard volume reconnection protocol to synchronize its state
with its new child. Thus, going to a new parent in the hierarchical
algorithm is no different than going to a server that has crashed and
lost a client's state in the original Volume Leases algorithm.
Similarly, to split the hierarchy, a child chooses a descendant of its
parent and starts using the new node as its parent, again using the
reconnection protocol to synchronize the state. For both
split and join, the decision to use a new parent can be made by
children at arbitrary times. The criteria for such decisions are a
matter of policy. Children can thus decide to find new parents to
improve fault tolerance or they can be told to use new parents to
improve performance.
Using join and split, an intermediate node failure is handled as
follows. If a node N cannot contact its parent P to renew a
lease, it sends the renewal message to one of its ancestors A,
triggering the volume reconnection protocol between N and A. Note
that if A cannot send an invalidation to P, it does not try to contact
N, but instead waits for the volume lease timeout; this means that
parents need to know only about their immediate children, not their
more distant descendents. Finally, when node P recovers, it can send
hints to its list of (former) children suggesting that they split from
A and join P instead.
For volumes with high read frequencies and many active
clients, a deep hierarchy can reduce read latency and distribute load.
However, for less popular objects, or for popular objects with low
read frequency, intermediate hops can increase read latency without
significantly reducing server load. Therefore, it is useful for
different volumes to construct different dynamic hierarchies. These
hierarchies can be constructed from the static hierarchy using the
split and join mechanisms in response to changing workloads. Hence, a
node can have different children in the static and dynamic
hierarchies: we refer to the former as static children, and to the
latter simply as children.
In the dynamic configuration algorithm a node N monitors the number of lease
requests it receives from its children and the fraction of these requests
that it can satisfy locally during time intervals of length T. Using this
data, N instructs its children to join with its parent if (1) the load from
its children would not cause the load on its parent to exceed a threshold
value and (2) its children would receive better read latency by skipping
N and going directly to the parent. N performs the latency
calculation as follows.
Let
RenewCost(N) be the cost for a child of N to renew a lease cached at
N, and let
RenewCost(P) be the cost for N to renew a lease cached at its
parent. If the fraction of renewals that N satisfies locally is
F, then the expected latency that a child of N pays to renew a
lease is
RenewCost(N) + (1-F) RenewCost(P). Assuming that the cost
of accessing N's
parent is about the same for both N and N's child, the expected
cost after a join is
RenewCost(P). When
RenewCost(N) + (1-F)
RenewCost(P) is greater than
RenewCost(P)
by some threshold, N instructs its children to perform a join unless
doing so would raise the load of the parent to an unacceptable level.
Similarly, to determine when to initiate a split, a node monitors the
requests from its children and initiates a split if (1) its local load
exceeds some threshold or (2) connecting a set of children to a
skipped node would reduce their expected read latency by some threshold.
A node N performs this read latency calculation by simulating the
performance of its skipped children as follows. For each static child
S, N maintains a simulated request count
ReqCount(S), hit count
HitCount(S), and volume lease expiration time VolExp(S). When a
child C of N contacts N to renew a lease, N updates the
statistics for the skipped child S that is a static ancestor of Cby (1) incrementing
ReqCount(S), (2) incrementing
HitCount(S) if
the current time is before VolExp(S), and (3) setting VolExp(S) to
the current time plus the volume lease length. Let
RenewCost(N) be
the cost for C to renew its lease at N and
RenewCost(S) be the
cost for C to renew its lease from the skipped child Sinstead. N tells C and its siblings to split from N and instead
use S as their parent if
.
Evaluation
We evaluate hierarchical consistency in three different deployment
configurations. First, we examine an aggressive deployment model,
generic hierarchy, to characterize the factors that affect the
behavior of the core algorithms and to determine the performance
limits of our approach. Second, we examine a simple clustered-server configuration in which the hierarchy is used to
distribute the algorithm across a LAN cluster in order to improve
scalability but not latency. This configuration might be used if a
service wishes to provide strong consistency for its data without
relying on having consistency-enabled intermediate proxies deployed
across the WAN. Third, we examine a server-proxy-client
configuration that maps well to infrastructure that is common today.
We evaluate these algorithms using simulations. To study scalability
and to evaluate how different aspects of workloads impact scalability,
we first use a series of synthetic workloads. We run each of these
experiments five times using different random seeds for workload
generation and show the 90% confidence interval for each point.
Then, to calibrate these
results, we examine a smaller, trace-based workload in the
context of the server-proxy-client configuration.
Based on these experiments, we reach the following conclusions:
- For the aggressive deployment scenario with flexible hierarchy
configurations, static hierarchies can reduce latency compared to
the flat Volume Leases algorithm for high request-rate services,
but they can increase latency for low request-rate services. In
contrast, the dynamic version always performs as well as the flat
algorithm for low request rates and as well as the static
hierarchy for high request rates.
- For workloads with modest request rates in the range of many
current web services, the flat Volume Leases algorithm with a
single server can scale to client populations in the tens or hundreds
of thousands of nodes; distributing the consistency algorithm
across a group of nodes--either in a cluster or across a
WAN--via hierarchies can provide scalability to millions of
clients even under aggressive workloads.
- In the server-proxy-client configuration, the simple static
hierarchy performs well for our web trace workload; this
configuration has the added benefit that it might also provide a
controlled way to traverse firewalls in order to deliver
consistency signals. The synthetic workload suggests that there
may be other workloads for which the dynamic algorithm's
flexibility is desirable.
Our methodology makes several significant assumptions and
simplifications. For our latency estimates, we do not simulate network
or server contention. We use a simple network topology and delay model
to make our analysis tractable. Finally, our default synthetic
workloads simulate one object per volume. This may understate the
apparent benefit of hierarchies because long-lived object leases are
much easier to cache in the hierarchy than short volume leases;
furthermore, the small number of objects per volume may also hurt the
relative performance of the static algorithm.
Our Generic Hierarchy configuration represents a system with
few constraints on deployment. We examine this
configuration to understand the behavior of the core algorithms
as we vary several key parameters. This configuration also models an
aggressive deployment strategy such as might be employed within a
large cache service or in a system where collections of servers and
cache systems coordinate to provide consistency.
The consistency hierarchy is a tree with one server at its root, Cclients at its leaves, and l-1 levels of intermediate nodes. We
designate the server to be the level 0 node of the consistency
hierarchy. For simplicity, we assume that at all levels of the tree
the degree d is the same, with dl = C. We defer the evaluation of
hierarchies with different fan-out at different levels for future work.
We use a simple cost model for accessing consistency servers. First,
we assume that all leaf nodes and internal nodes within a subtree
experience the same latency when they renew a lease with the root of
that subtree. Second, we assume that the latency experienced within a
subtree increases with the number of leaves in the subtree as follows:
subtrees with 100 or fewer leaves have a latency of 30 ms, subtrees
with 10,000 leaves have a latency of 100 ms, and subtrees with more
than 100,000 leaves have a latency of 400 ms; latencies for subtrees
with 100-10,000 nodes and 10,000 to 100,000 nodes are estimated
through interpolation. These latencies are meant to be suggestive of
department-, enterprise-, and Internet-scale delays, but do not
represent any specific system.
We use a synthetic workload and compute the average read latency and
server load when we simulate the accesses of a collection of clients
to a single volume. Out of Ntotal clients, we choose a subset of
clients of size
Nactive that access the volume with per-client
inter-access times determined using an exponential distribution around
an average value tread, which is expressed as a ratio of the average
inter-access time to the volume lease renewal time. In our initial
experiment, each volume contains a single object; we relax this
assumption later in this section.
A server's read frequency has a large impact on the performance of
hierarchical consistency. The higher the collective read frequency of
the clients below a proxy, the more often the proxy holds the
lease. Hence, if the read frequency is high the lease hit ratio will
be high and the proxy
can reduce read latency. Otherwise, if the collective read
frequency is low, then the lease hit ratio will be low.
Figure 1:
Average read latency as the per-client read
frequency is varied for a hierarchy of one million clients, of which 200,000
access the volume in question.
|
Figure 2:
Average read latency as the number of active clients varies
for a hierarchy of one million clients, each issuing requests to a
volume at a rate of 0.1 requests per volume lease period.
|
Figure 1 shows the average lease renewal latency as
the per-client read frequency is varied.
Figure 2
shows lease renewal latency as the fraction of clients that access the
volume in question is varied. The graphs compare the performance of a
flat, 2-level, 3-level, and 4-level consistency
hierarchy with part (a) of each figure showing performance for the
static algorithm and part (b) showing performance for the dynamic
algorithm. Figures 1 and 2
have the same general
shape because as one moves to the right
along the x axis the total request rate increases in both sets of
graphs. But, these
graphs represent different dimensions of the design space.
Read latency generally decreases as read frequency increases.
For high request rates,
the read latency falls even for the flat configuration because a
client issues multiple reads
within the period the client's volume lease is valid.
To interpret these graphs it is helpful to consider where different
classes of services might lie or where a single service might lie
under different workloads. For example, a weather service that is
visited by an average client once a day for one minute and that uses a
10-second lease period would correspond to a read frequency of less
than 0.001 reads per volume lease period per client. Similarly, a news
service whose typical users visit for 5 minutes during the 8-hour
working day would correspond to a volume renewal frequency near 0.01
per volume lease period per client. The read frequency of that same
service might jump above 0.1 or even near 1 for periods of time during
news events of widespread interest as clients constantly monitor the
news for new developments. Similarly, emerging program-driven
applications might span a wide range of the parameter space.
With respect to lease renewal latency as a function of read frequency,
the main observations are as follows:
- Hierarchies can significantly reduce latency for active and
popular services.
- The dynamic hierarchy succeeds in matching the latency of
the flat Volume Leases algorithm for less active or less popular
services while matching the performance of the static hierarchy for
busier services. Relative to flat Volume Leases, the static hierarchy
can hurt latency for less active
or less popular services but can help latency for active and popular
services.
- The dynamic hierarchy appears to be a good default choice for
this configuration. If a service's access patterns are known and
if these access patterns do not change much, then
either flat Volume Leases or a static hierarchy may be
reasonable, depending on the workload.
Finally, note that the variations among different depths of underlying
static trees depend both on interactions between the
number of clients under each level of a subtree and on our assumptions
on the network distances between subtrees as a function of subtree
size. Hence, this experiment should not be used for general comparisons
between the number of levels that should be used in the underlying
hierarchy.
Figure 3:
Average read latency as the per-client read
frequency is varied for a hierarchy of 100,000, of which 20,000
access the volume in question.
|
Figure 4:
Average server load for handling renewal requests as the per-client read
frequency is varied for a hierarchy of one million clients, of which 200,000
access the volume in question.
|
Figure 3 shows similar
experiments but with 100,000 total clients (20,000 of them active)
rather than 1,000,000. Comparing these results to those
with more clients provides intuition about the effects of
scaling the client population, which may help predict system behavior
for populations larger than the 1,000,000 that we are able to simulate.
- As expected, increasing (decreasing) the total number of clients
decreases (increases) the per-client request rate for which
hierarchies begin to pay off relative to the flat Volume Leases
configuration. We observe similar results when we vary the number of
active clients (graph omitted).
Figure 4
shows how server load varies with client request rate hierarchies
spanning one million clients. (Results for varying the number of
active clients or simulating a universe of 100,000 clients are
omitted, but are qualitatively similar). Assuming that a server can
handle a few thousand requests per volume lease period, we conclude:
- The flat Volume Leases algorithm scales to tens of thousands
of clients under workloads corresponding to a range of reasonable web
access patterns.
- The addition of hierarchies supports scalability to many
millions of clients under nearly arbitrary workloads because it bounds
the rate of requests at the root to one request per volume lease
period per immediate child of the root.
To make simulating a large scale hierarchy feasible, we have so far
considered only the cost of renewing volume leases. We have not
examined the cost of renewing or invalidating object leases. This
simplification affects our results in two ways. First, it causes us
to understate average read time because in reality clients
will sometimes have valid volume leases but still need to fetch object
leases. This effect should be modest because object leases are much
longer than volume leases. Second, this simplification may cause us
to understate the benefit of consistency proxies, particularly when
read frequency is low, because consistency proxies can cache
long object leases more effectively than short volume leases. To calibrate
the effect of object leases for popular servers, we run several
simulations with multiple object leases per volume. The results are
illustrated in
Figures 5 to 10. Due
to space constraints, we show the graphs for the dynamic hierarchy
algorithm and omit those for the static algorithm; the static results differ
little from the dynamic ones.
Figure 5:
Average read latency under the dynamic algorithm as the write
frequency for 10 objects in a server volume
is varied. Average read frequency per client is fixed at 0.01 read per
client per volume lease period.
|
Figure 6:
Same as Figure 5, except that the read frequency is
0.1 read per client per volume lease period.
|
Figure 7:
Average server messages per volume lease period under the dynamic
algorithm as the write frequency
for 10 objects in a server volume is varied. Average read frequency
per client is fixed at 0.01 read per client per volume lease period.
|
Figure 8:
Same as Figure 7, except that the read frequency per
client is 0.1 read per client per volume lease period.
|
In these experiments, the server volume contains 10 objects. Each
object is modified independently with average write frequency varying
from 0.1 writes to 1000 writes per client read. We illustrate
performance for servers that have average 0.01 read and 0.1 read per
volume lease per client. For example, a system with 30 second volume
leases, 3000 seconds between client reads, and 300 seconds between
writes would correspond to the Write Frequency = 10 point in
Figure 5.
Figure 5 shows the average read latency as the
write frequency changes. Each client issues an average of 0.01 reads
per volume lease period. When write frequency is between 0.1 to 10
writes per client read, the results closely match our simplified
experiment. Only after write frequency gets higher than 10
writes per client read does the read latency increase become
noticeable. Figure 6 is similar to
Figure 5, except the read frequency is set to
0.1 reads per volume lease period instead of
0.01. Figures 7 and
8 show the average server load under
the same workload as Figures 5 and
6.
Figure 9:
Average write latency seen by server for the dynamic algorithm as the
write frequency
for 10 objects in a server volume is varied. Average read frequency
per client is fixed at 0.01 read per client per volume lease period.
|
Figure 10:
Same as Figure 9, except that the read frequency per
client is 0.1 read per client per volume lease period.
|
Figures 9 and 10 show
the average write latency as the write frequency changes. We calculate
the write latency by finding the critical path from when the server
sends its first invalidation until it receives the final reply. At
each node, N, of the hierarchy, we charge
where
nValidChildren(N) is the number of N's direct children that have
valid volume leases. Note that by using the delayed invalidation
optimization [20], the sending of invalidation messages to nodes
whose volume leases have expired can be removed from the critical
path. The latency
to a child is determined by the topology according to our standard
formula, and the cost per child is set to 1 ms.
The data indicate three main effects:
- When write frequency increases, the benefit of hierarchy for
reads is eroded.
- As write frequency decreases, the write latency increases. This is
because the number of valid leases that accumulate between writes, and
thus must be invalidated, increases.
- For a frequently
accessed popular server, flat volume leases can introduce significant
write delays, but hierarchies can remedy this problem.
The hierarchical consistency mechanisms can be used not only to
distribute consistency algorithms across a WAN, but also to split a
consistency service across a clustered web server on a local area
network (LAN) or system area network (SAN). Although an algorithm
optimized for splitting consistency state
and load across a cluster with a fast network might marginally
outperform our more general mechanisms, such an algorithm must solve
the same basic problems of fault tolerance, distributing
invalidations, gathering acknowledgments, and partitioning state that
our algorithm handles, so the simplicity of using a single framework
for both LAN and WAN distribution appears attractive.
Figure 11:
Server lease renewal load as the per-client read
frequency is varied for a static server cluster hierarchy serving one million
clients, of which 200,000
access the volume in question.
|
Figure 11 shows the load on the server in the server
cluster hierarchy where the server and all of the internal nodes of
the consistency hierarchy are located in a tightly-coupled cluster,
and the lowest internal nodes in the hierarchy communicate across a
WAN with the clients. This configuration is not designed to improve
latency, just load-scalability. As a result, read latency cannot be
affected significantly by modifying the consistency structure. Hence,
it is not necessary to build a dynamic hierarchy in this circumstance.
Based on this experiment, we conclude:
- For the server-cluster configuration, the static hierarchy (with
split and join for fault tolerance) provides a simple mechanism to
scale the flat volume leases algorithm by distributing it across a
group of nodes in a cluster; dynamic configuration to minimize latency
is not required.
Server-proxy-client
Figure 12:
Average read latency as the per-client read
frequency is varied for a server-proxy-client hierarchy of one million
clients, of which 200,000
access the volume in question. In the server-proxy-client hierarchy the
internal nodes in the consistency hierarchy are all proxies serving
10,000 clients each.
|
Figure 13:
Server lease renewal load as the per-client read
frequency is varied for a server-proxy-client hierarchy serving one million
clients, of which 200,000
access the volume in question.
|
Figures 12 and 13 show the
latency and load measurements when the hierarchy algorithms are run on
the server-proxy-client underlying hierarchy with one million clients
grouped into 100 proxy-groups of 10,000
clients per group. This experiment suggests two points:
- For low read frequencies, the dynamic hierarchy where clients
may contact servers directly has a modest advantage over the static
hierarchy.
- At high read frequencies both the static and dynamic
configurations significantly outperform the flat configuration.
Figure 14:
Average read latency and fraction of renewal requests sent to the
server for the four medium-loaded and four
heavily-loaded volumes from the DEC trace workload under a
server/proxy/client hierarchy in which the
internal node in the consistency hierarchy is the proxy serving the
DEC clients.
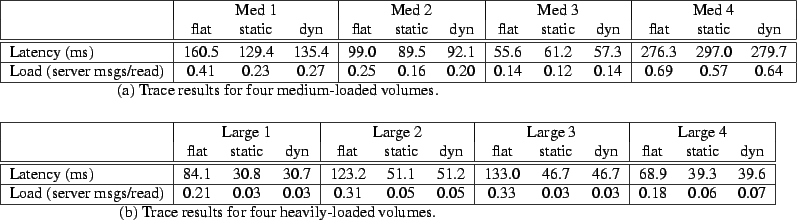 |
Figure 14 shows latency for several selected volumes
under a trace workload. The workload is the DEC
trace [4], and we configure the system with all
clients under a single proxy. We map each server in the trace to a
different volume. We present results for 8 selected servers: the 4 most popular
ones and 4 of medium popularity.
- The trace workloads include multiple objects per volume, and long
object leases are easier to cache in a hierarchy. As a result, the
static hierarchy begins to pay dividends even with relatively low
access rates.
This suggests that for many current web workloads, the simple static
hierarchy using the simple server-proxy-client hierarchy may be a
reasonable deployment option. This configuration might also provide a
practical way to traverse firewalls to deliver consistency signals.
In previous work, we compared non-hierarchical consistency algorithms
based on volume leases to traditional callback and polling algorithms.
We found that algorithms based on volume leases could both (i)
significantly outperform traditional callback or object lease
algorithms for a given maximum tolerable server write delay; and (ii)
could provide stronger consistency guarantees with performance
competitive with callback-based algorithms. Earlier studies by
Gwertzman and Seltzer [8] and by Liu and
Cao [13] also compare callbacks to polling. Liu and Cao
find the performance of the two approaches to be competitive.
Gwertzman and Seltzer find that polling with adaptive timeouts can
outperform callbacks, but that to gain this advantage the polling
algorithm may return stale data more than 4% of the time.
Worrell [19] compares callback and polling protocols in a
hierarchical caching system and concludes that the callback algorithms
have performance competitive with polling for reasonable time-out
values.
Yu et. al [21] independently develop a consistency scheme based
on hierarchy, leases, and volumes; this proposal shares many
properties with ours, but it differs from ours in three main ways.
First,
it places a bound on object staleness, whereas our
algorithm can be used either to provide
strong consistency or to bound staleness [20].
Second, its reconnection protocol requires a client that becomes
disconnected to discard all volume and object leases and renew them
individually.
Third, its consistency
servers periodically multicast volume lease
renewals and recent object invalidations to their children. Compared
to client-initiated volume lease renewal, their approach ``pushes''
renewals to clients that are not currently accessing a volume; it
may thus improve read latency while increasing network traffic and
client overheads. Beyond these algorithmic differences, the
experimental focus of the study complements ours. Yu et. al primarily
focus on comparing the performance of hierarchical invalidation to
polling, whereas we focus on understanding the scalability properties
of hierarchies.
Cohen et. al [3] study the use of volumes for prefetching and
consistency. The consistency algorithms they examine are
best-effort algorithms based on client polling. Some of their
prefetching techniques might also be useful for ``prefetching'' volume
lease renewals in our system. We speculate that adding such
prefetching to our system would reduce the read latency cost of
hierarchies but magnify the value of hierarchies in reducing server
load. Exploring this combination appears to be an interesting area
for further study.
Krishnamurthy and Wills [12] examine ways to improve
polling-based consistency by piggy-backing optional invalidation
messages on other traffic between a client and server. Our
volume-based approach allows delayed invalidations [20]
where servers delay object invalidation messages to clients whose
volume leases have expired. Combining delayed invalidations with
piggy-backing may be another useful optimization.
Cache consistency protocols have long been studied for distributed
file systems [9,15,17]. Several aspects
of Coda's [11] consistency protocol are reflected in our
algorithms. In particular, our notion of a volume is similar to that
used in Coda [14]. However, ours differ in two key
respects. First, Coda does not associate volumes with leases, and
relies instead on other methods to determine when servers and clients
become disconnected. Second, because Coda is designed for different
workloads, its design trade-offs are different. For example, because
Coda expects clients to communicate with a small number of servers and
it regards disconnection as a common occurrence, Coda aggressively
attempts to set up volume callbacks to all servers on each hoard walk.
Our reconnection protocol in which clients help servers recover the state
they need is based on the server crash recovery protocol in
Sprite [1].
Finally, we note that Volume Leases on the set of all objects provided
by a server can be thought of as providing a framework for the
``heartbeat'' messages used in many distributed state systems.
In this paper we have shown that the Volume Leases algorithm can provide
strong consistency for Internet services with hundreds of thousands of
clients. We have also shown how the Volume Leases can be applied to
hierarchical caches to perform well for workloads with millions of clients.
The key mechanisms, join and split, can be implemented using a simple
extension of the Volume Leases algorithm. Finally, we have evaluated a
number of hierarchy configurations, and our results show that a dynamically
configurable hierarchy provides tremendous amounts of scalability.
We thank the anonymous USITS reviewers and our shepherd, Peter
Honeyman, for their valuable feedback on earlier drafts of this work.
- 1
-
M. Baker.
Fast Crash Recovery in Distributed File Systems.
PhD thesis, University of California at Berkeley, 1994.
- 2
-
A. Chankhunthod, P. Danzig, C. Neerdaels, M. Schwartz, and K. Worrell.
A Hierarchical Internet Object Cache.
In Proc. of the 1996 USENIX Technical Conf., January 1996.
- 3
-
E. Cohen, B. Krishnamurthy, and J. Rexford.
Improving End-to-End Performance of the Web Using Server Volumes and
Proxy Filters.
In Proc. of the ACM SIGCOMM '98 Conf. on Applications,
Technologies, Architectures, and Protocols for Computer Communication, 1998.
- 4
-
Digital Equipment Corporation.
Digital's Web Proxy Traces.
ftp://ftp.digital.com/pub/DEC/traces/proxy/webtraces.html,
September 1996.
- 5
-
B. Duska, D. Marwood, and M. Feeley.
The Measured Access Characteristics of World-Wide-Web Client Proxy
Caches.
In Proc. of the USENIX Symposium on Internet Technologies and
Systems, December 1997.
- 6
-
S. Gadde, J. Chase, and M. Rabinovich.
Directory Structures for Scalable Internet Caches.
Technical Report CS-1997-18, Duke University Department of Computer
Science, November 1997.
- 7
-
C. Gray and D. Cheriton.
Leases: An Efficient Fault-Tolerant Mechanism for Distributed File
Cache Consistency.
In Proc. of the 12th ACM Symposium on Operating Systems
Principles, pages 202-210, 1989.
- 8
-
J. Gwertzman and M. Seltzer.
World-Wide Web Cache Consistency.
In Proc. of the 1996 USENIX Technical Conf., January 1996.
- 9
-
J. Howard, M. Kazar, S. Menees, D. Nichols, M. Satyanarayanan, R. Sidebotham,
and M. West.
Scale and Performance in a Distributed File System.
ACM Trans. on Computer Systems, 6(1):51-81, February 1988.
- 10
-
Kermarrec, Kuz, van Steen, and Tanenbaum.
A Framework for Consistent, Replicated Web Objects.
In Proc. of the 18th Intl. Conf. on Distributed Computing
Systems, 1998.
- 11
-
J. Kistler and M. Satyanarayanan.
Disconnected Operation in the Coda File System.
ACM Trans. on Computer Systems, 10(1):3-25, February 1992.
- 12
-
B. Krishnamurthy and C. Wills.
Piggyback Server Invalidation for Proxy Cache Coherency.
In Proc. of the 7th Intl. World Wide Web Conf., 1998.
- 13
-
C. Liu and P. Cao.
Maintaining Strong Cache Consistency in the World-Wide Web.
In Proc. of the Seventeenth Intl. Conf. on Distributed Computing
Systems, May 1997.
- 14
-
L. Mummert and M. Satyanarayanan.
Large Granularity Cache Coherence for Intermittent Connectivity.
In Proc. of the Summer 1994 USENIX Conf., June 1994.
- 15
-
M. Nelson, B. Welch, and J. Ousterhout.
Caching in the Sprite Network File System.
ACM Trans. on Computer Systems, 6(1), February 1988.
- 16
-
C. Plaxton, R. Rajaram, and A. Richa.
Accessing nearby copies of replicated objects in a distributed
environment.
In Proc. of the 9th Annual ACM Symposium on Parallel Algorithms
and Architectures, pages 311-320, June 1997.
- 17
-
V. Srinivasan and J. Mogul.
Spritely NFS: Experiments with Cache Consistency Protocols.
In Proc. of the 12th ACM Symposium on Operating Systems
Principles, pages 45-57, December 1989.
- 18
-
R. Tewari, M. Dahlin, H. Vin, and J. Kay.
Design Considerations for Distributed Caching on the Internet.
In Proc. of the 19th Intl. Conf. on Distributed Computing
Systems, May 1999.
- 19
-
K. Worrell.
Invalidation in Large Scale Network Object Caches.
Master's thesis, University of Colorado, Boulder, 1994.
- 20
-
J. Yin, L. Alvisi, M. Dahlin, and C. Lin.
Using Leases to Support Server-Driven Consistency in Large-Scale
Systems.
In Proc. of the 18th Intl. Conf. on Distributed Computing
Systems, May 1998.
- 21
-
H. Yu, L. Breslau, and S. Schenker.
A Scalable Web Cache Consistency Architecture.
In Proc. of the ACM SIGCOMM '98 Conf. on Applications,
Technologies, Architectures, and Protocols for Computer Communication,
September 1999.
Hierarchical Cache Consistency in a WAN1
This document was generated using the
LaTeX2HTML translator Version 98.1p1 release (March 2nd, 1998)
Copyright © 1993, 1994, 1995, 1996, 1997,
Nikos Drakos,
Computer Based Learning Unit, University of Leeds.
The command line arguments were:
latex2html -split 0 -toc_depth 0 -no_navigation -antialias head.tex.
The translation was initiated by Jian Yin on 1999-08-30
Footnotes
- ... WAN1
- This work was supported in part by
an NSF Research Infrastructure Award CDA-9624082, DARPA/SPAWAR grant
number N66001-98-8911, and grants from
Dell, Novell, and Sun. Dahlin and Alvisi were
also supported by NSF CAREER grants CCR-9733842 and CCR-9734185,
respectively.
Jian Yin
1999-08-30
|