USITS '03 Paper   
[USITS '03 Tech Program Index]
Pp. 71-85 of the Proceedings |  |
Conflict-Aware Scheduling for Dynamic Content Applications
Abstract:
We present a new lazy replication technique, suitable for scaling the
back-end database of a dynamic content site using a cluster
of commodity computers.
Our technique, called conflict-aware scheduling, provides
both throughput scaling and 1-copy serializability.
It has generally been believed that this combination
is hard to achieve through replication
because of the growth of the number of conflicts.
We take advantage of the presence in a database cluster of a scheduler
through which all incoming requests pass.
We require that transactions specify the tables
that they access at the beginning of the transaction.
Using that information, a conflict-aware scheduler relies on
a sequence-numbering scheme to implement 1-copy serializability, and
directs incoming queries in such a way that the number of conflicts is
reduced.
We evaluate conflict-aware scheduling using the TPC-W e-commerce benchmark.
For small clusters of up to eight database replicas, our evaluation is
performed through measurements of a web site implementing the TPC-W
specification. We use simulation to extend our measurement
results to larger clusters, faster database engines, and lower
conflict rates.
Our results show that conflict-awareness brings considerable benefits
compared to both eager and conflict-oblivious lazy replication
for a large range of cluster sizes, database speeds, and conflict rates.
Conflict-aware scheduling provides near-linear throughput scaling up to
a large number of database replicas for the browsing and shopping workloads
of TPC-W.
For the write-heavy ordering workload, throughput scales, but only
to a smaller number of replicas.
1 Introduction
This paper studies replication in database
clusters [12,17,24] serving
as back-ends in dynamic content sites.
Dynamic content sites commonly use a three-tier architecture, consisting
of a front-end web server, an
application server implementing the business logic of the site, and a
back-end database (see Figure 1).
The (dynamic) content of the site is stored in the database.
Figure 1:
Common Architecture for Dynamic Content Sites
 |
We focus in this paper on the case where the database back-end is the
bottleneck.
Practical examples of such situations may be found in e-commerce
sites [1] or bulletin boards [19].
Bottlenecks in the web server tier are easily addressed by replicating
the web server.
Since it serves only static content, replication
in this tier does not introduce any consistency issues.
Addressing bottlenecks in the application server is a subject of further
study.
Rather than following the common approach of using a very expensive
high-end database machine as the back-end [6,11],
we investigate an alternative approach using
a cluster of low-cost commodity machines.
As Figure 2 shows, a scheduler
is interposed between the application server(s) and the database cluster.
The scheduler virtualizes the database cluster and makes it look to the
application server as a single database.
Therefore, no changes are required in the application server.
Similarly, the methods presented in this paper do not require any
changes to the database engine.
Figure 2:
Basic Cluster Architecture
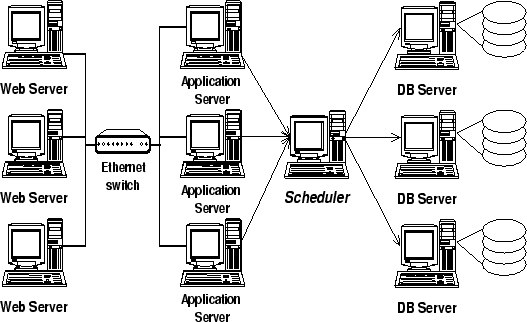 |
Based on observing the workload imposed on the database by dynamic
content sites [1], and in particular by TPC-W [22],
we argue that the nature of e-commerce workloads is such that
lazy read-one, write-all replication [23] is the preferred
way to distribute the data across the cluster.
Furthermore, we argue that strong consistency (in technical terms,
1-copy serializability [4]) is desirable
between the database replicas.
From the combination of these two requirements - lazy replication and
1-copy serializability - stems the challenge addressed by this paper.
Lazy replication algorithms asynchronously propagate replica updates
to other nodes, possibly even after the updating transaction commits.
They do not provide 1-copy serializability, since, for
instance, writes may be propagated in different orders to different
replicas.
Past approaches have used reconciliation to achieve eventual
replica consistency. Predictions of the number of reconciliations
with a large number of replicas have, however,
been discouraging [8]. More recent work [12]
suggests that for small clusters,
both 1-copy-serializability and acceptable performance can
be achieved by aborting conflicting transactions.
Our goal is also to use lazy replication and achieve 1-copy
serializability,
but instead of resolving conflicts by aborting conflicting
transactions, we augment the scheduler (see
Figure 2)
with a sequence numbering scheme to guarantee 1-copy serializability.
To improve performance we also augment the scheduler to be conflict-aware.
Intuitively, a scheduler is conflict-aware if it directs incoming
requests in such a way that the time waiting for conflicts is reduced.
For instance, a write on a data item may have been sent to all replicas,
but it may have completed only at a subset of them.
A conflict-aware scheduler keeps track of the completion status of each
of the writes.
Using this knowledge, it sends a conflicting read that
needs to happen after this write to a replica where it knows the write
has already completed.
A concern with a conflict-aware scheduler is the extra state
and the extra computation in the scheduler, raising the possibility
of it becoming the bottleneck
or making it an issue in terms of availability and fault tolerance
of the overall cluster.
We present a lightweight implementation of a conflict-aware scheduler,
that allows a single scheduler to support a large number of databases.
We also demonstrate how to replicate the scheduler for availability and
increased scalability.
Our implementation uses common software platforms such as
the Apache Web server [3], the PHP scripting
language [16],
and the MySQL relational database [14].
As we are most interested in e-commerce sites,
we use the TPC-W benchmark in our evaluation [22].
This benchmark specifies three workloads (browsing, shopping and
ordering) with different percentages of writes in the workload.
Our evaluation uses measurement of the implementation for small
clusters (up to 8 database machines).
We further use simulation to assess the performance effects of
larger clusters, more powerful database machines, and varying
conflict rates.
Finally, in order to provide a better understanding of the performance
improvements achieved by a conflict-aware scheduler, we have implemented
a number of alternative schedulers that include only some of the features of
the
strategy implemented in conflict-aware scheduling.
Our results show that:
- The browsing and shopping workloads scale very well, with close to
linear increases in throughput up to 60 and 40 databases respectively.
The ordering workload scales only to 16 databases.
- The benefits of conflict awareness in the scheduler are substantial.
Compared to a lazy protocol without this optimization, the
improvements are up to a factor of 2 on our
largest experimental platform, and up to a factor of 3 in the
simulations.
Compared to an eager protocol, the improvements
are even higher (up to a factor of 3.5 in the experiments, and
up to a factor of 4.4 in the simulations).
- Simulations of more powerful database machines and varying
conflict rates validate the performance advantages of conflict-aware
scheduling on a range of software/hardware environments.
- One scheduler is enough to support scaling for up to 60
MySQL engines for all workloads, assuming equivalent nodes are used
for the scheduler and MySQL engine. Three schedulers are necessary
if the databases become four times faster.
- The cost of maintaining the extra state in the scheduler is minimal
in terms of scaling, availability and fault tolerance.
- Even with conflict avoidance, the eventual scaling limitations stem
from conflicts.
The outline of the rest of the paper is as follows.
Section 2 provides the necessary background
on the characteristics of dynamic content applications.
Section 3 introduces our solution.
Section 4
describes our prototype implementation.
Section 5 describes the fault tolerance
aspects of our solution.
Section 6
describes other scheduling techniques introduced for
comparison with a conflict-aware scheduler.
Section 7 presents our benchmark and
experimental platform.
We investigate scaling experimentally
in Section 8, and by simulation in
Section 9.
Section 10 discusses related work.
Section 11 concludes the paper.
2 Background and Motivation
In this section we provide an intuitive motivation for the methods used
in this paper.
This motivation is based on observing the characteristics of the
workloads imposed
on the database of a dynamic content site by a number of
benchmarks [1],
in particular by TPC-W [22].
First, there is a high degree of locality in the database access
patterns.
At a particular point in time,
a relatively small working set captures a large fraction of the
accesses.
For instance, in an online bookstore, best-sellers are accessed very
frequently.
Similarly, the stories of the day on a bulletin board, or the active
auctions on an auction site receive the most attention.
With common application sizes, much of the working set can be captured
in memory.
As a result, disk I/O is limited.
This characteristic favors replication as a method for distributing the
data compared to alternative methods such as data partitioning.
Replication is suitable to relieve hot spots, while data partitioning is
more suitable for relieving high I/O demands [5].
Replication brings with it the need to define a consistency model for
the replicated data.
For e-commerce sites, 1-copy serializability appears
appropriate [4].
With 1-copy serializability conflicting operations of different
transactions appear in the same total order at all replicas.
Enforcing 1-copy serializability avoids, for instance, a scenario
produced by re-ordering of writes on different replicas in which
on one replica it appears that one
customer bought a particular item, while on a different replica it
appears that another customer bought the same item.
Second, the computational cost of read queries is typically much larger
than that of update queries.
A typical update query consists of updating a single record
selected by an equality test on a customer name or a product identifier.
In contrast, read queries may involve complex search criteria
involving many records, for instance "show me the ten most popular books on
a particular topic that have been published after a certain date".
This characteristic favors read-one, write-all replication: the
expensive components of the workloads (reads) are executed on a single
machine,
and only the inexpensive components (writes) need to be executed on all
machines.
The desirability of read-one, write-all over more complex majority-based
schemes has also been demonstrated under other
workloads [12,24].
Third, while locality of access is beneficial in terms of keeping data
in memory, in a transactional setting it has the potential drawback of
generating frequent conflicts, with the attendant cost of waiting for
conflicts to
be resolved.
The long-running transactions present in the typical
workloads may cause conflicts to persist for a long time.
The frequency of conflicts also dramatically increases as a result of
replication [8].
Frequent conflicts favor lazy replication methods, that
asynchronously propagate updates [17,21,24].
Consider, for instance, a single-write transaction followed by a
single-read transaction. If the two conflict, then in a conventional
synchronous update protocol, the read needs to wait until the write
has completed at all replicas. In contrast, in a lazy update protocol,
the read can proceed as soon as the write has executed at its replica.
If the two do not conflict, the read can execute in parallel with the
write, and the benefits of asynchrony are much diminished.
Finally, frequent conflicts lead to increased potential for deadlock.
This suggests the choice of a concurrency control method that avoids
deadlock.
In particular, we use conservative two-phase
locking [4], in which all locks
are acquired at the beginning of a transaction.
Locks are held until the end of the transaction.
In summary, we argue that lazy read-one, write-all replication in
combination with conservative two-phase locking is suitable
for distributing data across a cluster of databases in a dynamic content
site.
In the next section, we show that by augmenting the scheduler with a
sequence numbering scheme, lazy replication can be extended to provide
1-copy serializability.
We also show how the scheduler can be extended to include
conflict awareness, with superior performance as a result.
3 Design
3.1 Programming Model
A single (client) web interaction may include one or more transactions,
and a single transaction may include one or more read or write
queries.
The application writer specifies where in the application code
transactions begin and end.
In the absence of transaction delimiters, each single query is
considered a transaction and is automatically committed (so
called "auto-commit" mode).
At the beginning of each transaction consisting of more than one query,
the application writer inserts a lock acquire specifying
all tables accessed in the transaction and their access types (read or
write).
This step is currently done by hand, but could be automated.
Locks for single-operation transactions do not need to be specified.
We consider a cluster architecture for a dynamic content site,
in which a scheduler distributes
incoming requests to a cluster of database replicas and delivers the
responses to the application servers (see
Figure 2).
The scheduler may itself be replicated for performance or for
availability.
The presence of the scheduler is transparent to the application server
and the database, both of which are unmodified.
If there is more than one scheduler in a particular
configuration, the application server is assigned a particular
scheduler at the beginning of a client web interaction.
This assignment is currently done by round-robin.
For each operation in a particular client web interaction, the
application server only
interacts with this single scheduler, unless the scheduler fails.
These interactions are synchronous: for each query, the execution of the
business logic for this
particular client web interaction in the application server waits
until it receives a response from the scheduler.
The application server sends the scheduler
lock requests for multiple-operation transactions,
reads, writes, commits and aborts.
When the scheduler receives a lock request, a write or a commit
from the application server,
it sends it to all replicas and returns the response as soon as it
receives a response from any of the replicas.
Reads are sent only to a single replica, and the response is
sent back to the the application server as soon as it is
received from that replica.
The scheduler maintains 1-copy serializability by assigning a unique
sequence number to each transaction.
This assignment is done at the beginning of the transaction.
For a multiple-operation transaction the sequence number is assigned
when that transaction's lock request arrives at the scheduler.
For a single-operation transaction, sequence number assignment is
done when the transaction arrives at the scheduler.
Lock requests are sent to all replicas, executed in order of their
assigned sequence numbers, and held until commit, thus forcing all
conflicting operations to execute in a total order identical at all
replicas, and thus enforcing 1-copy serializability.
Transactions consisting of a single read query are treated differently.
A single-read transaction holds locks only on the single replica
where it executes.
This optimization results in a very substantial performance
improvement without violating 1-copy serializability.
Due to the asynchrony of replication,
at any given point, some replicas may have fallen behind with the
application of writes. Furthermore, some replicas may have not been able
to acquire the locks for a particular transaction, due to conflicts.
For reads, other than reads in single-read transactions, the scheduler
first determines the set of replicas where the locks for its
enclosing transaction have been acquired and where all previous
writes in the transaction have completed.
It then selects the least loaded replica from this set as the replica
to receive the read query.
The scheduler tries to find conflict-free replicas for single-read
transactions as well, but may not be able to find one.
Conflict-aware scheduling requires that the scheduler maintains
the completion status of lock requests and writes,
for all database replicas.
4 Implementation
The implementation consists of three types of processes:
scheduler processes (one per scheduler machine),
a sequencer process (one for the entire cluster),
and database proxy processes (one for each database replica).
The sequencer assigns
a unique sequence number to each transaction and thereby implicitly
to each of its locks.
A database proxy regulates access to its database server
by letting an operation proceed only if the database has already
processed
all conflicting operations that precede it in sequence number order and
all operations that precede it in the same transaction.
The schedulers form the core of the implementation.
They receive the various operations from the application servers,
forward them to one or more of the database proxies,
and relay the responses back to the application servers.
The schedulers also interact with the sequencer to obtain
a sequence number for each transaction.
In the following, we describe the state maintained at the scheduler
and at the database proxy to support failure-free execution, and the
protocol steps executed on receipt of each type of operation and its
response.
Additional state maintained for fault-tolerance purposes is described
in Section 5.
The scheduler maintains for each active transaction its
sequence number and the locks requested by that transaction.
In addition, it maintains a record for each operation that is
outstanding with one or more database proxies.
A record is created when an operation is received from the application
server, and updated when it is sent to the database engines, or when a reply
is received from one of them.
The record for a read operation is deleted as soon as the response
is received and delivered to the application server.
For every replicated operation (i.e., lock request, write, commit
or abort), the corresponding record is deleted only when all
databases have returned a response.
The scheduler records the current load of
each database (see section 4.10).
This value is updated with new information included in
each reply from a database proxy.
The database proxy maintains a reader-writer lock [23] queue
for each table.
These lock queues are maintained in order of sequence numbers.
Furthermore, the database proxy maintains
transaction queues, one per transaction, and
an out-of-order queue for all operations that arrive out of sequence number
order.
For each transaction queue, a head-of-queue record maintains the current
number of locks granted to that transaction.
Each transaction queue record maintains the operation to be
executed. Transaction queue records are maintained in order
of arrival.
4.4 Lock Request
For each lock request,
the scheduler obtains a sequence number
from the sequencer and stores this information together with the
locks requested for the length of the transaction.
The scheduler then tags the lock request with its sequence
number and sends it to all database proxies.
Each database proxy executes the lock request locally and
returns an answer to the scheduler when the lock request is granted.
The lock request is not forwarded to the database engine.
A lock request that arrives at the database proxy in sequence number order is
split into separate requests for each of the locks requested.
When all locks for a particular transaction have been granted, the proxy
responds to the scheduler.
The scheduler updates its record for that transaction, and
responds to the application server, if this is the first response
to the lock request for that transaction.
A lock request that arrives out-of-order (i.e., does not have a sequence
number that is one more than the last processed lock request)
is put in the out-of-order queue.
Upon further lock request arrivals, the proxy checks whether any request
from the out-of-order queue can now be processed.
If so, that request is removed from the out-of-order queue and processed
as described above.
4.5 Reads and Writes
As the application executes the transaction, it sends read and
write operations to the scheduler.
The scheduler tags each operation with
the sequence number that was assigned to the transaction.
It then sends write operations to all database proxies, while reads
are sent to only one database proxy.
The scheduler sends each read query to one of the replicas where the
lock request and the previous writes of the enclosing transaction have
completed.
If more than one such replica exists, the
scheduler picks the replica with the lowest load.
The database proxy forwards a read or write operation
to its database only when all previous operations
in the same transaction (including lock requests) have been executed.
If an operation is not ready to execute, it is queued in the
corresponding transaction queue.
On the completion of a read or a write at the database,
the database proxy receives the response and forwards it
to the scheduler.
The proxy then submits the next operation waiting in the
transaction queue, if any.
The scheduler returns the response to the application server if this is
the first response it received for a write query or if it is
the response to a read query.
Upon receiving a response for a write from a database proxy,
the scheduler updates its corresponding record to reflect the reply.
The scheduler tags the commit/abort received from the application server
with the sequence number and locks requested at the start of the
corresponding transaction, and forwards the commit/abort to all replicas.
If other operations from this transaction are pending in the
transaction queue, the commit/abort is inserted at the tail of
the queue.
Otherwise, it is submitted to the database.
Upon completion of the operation at the database, the database proxy
releases each lock held by the transaction, and
checks whether any lock requests in the queues can be granted
as a result.
Finally, it forwards the response to the scheduler.
Upon receiving a response from a database proxy,
the scheduler updates the corresponding record to reflect the reply.
If this is the first reply, the scheduler
forwards the response to the application server.
The read is forwarded to a database proxy, where it
executes after previous conflicting transactions have finished.
In particular, requests for individual locks are queued in the
corresponding lock queues, as with any other transaction, and
the transaction is executed when all of its locks are available.
To choose a replica for the read, the scheduler first selects the set
of replicas where the earlier update transactions in the same client web
interaction, if any, have completed.
It then determines the subset of this set at which no conflicting locks
are held. This set may be empty.
It selects a replica with the lowest load in the latter set, if it is
not empty, and otherwise from the former set.
Single-update transactions are logically equivalent to multiple-operation
transactions, but in the implementation they need to be treated a little
differently; the necessary
locks are not specified by the application logic, hence
there is no explicit lock request.
When the scheduler receives a single-update transaction, it computes the
necessary locks and obtains a sequence number for the transaction.
The transaction is then forwarded to all replicas with that additional
information. Thus, the lock request is implicit rather than sent in
a separate lock request message to the database proxy, but
otherwise the database proxy treats a single-update transaction in the
same way as any multiple-update transaction.
4.10 Load Balancing
We use the Shortest Execution Length First (SELF) load balancing
algorithm.
We measure off-line the execution time of each query on an idle machine.
At run-time, the scheduler estimates the load on a replica as the sum of
the (apriori measured) execution times of all queries outstanding
on that back-end.
SELF tries to take into account
the widely varying execution times for different query types.
The scheduler updates the load estimate for each replica with feedback
provided by the database proxy in each reply.
We have shown elsewhere [2] that SELF outperforms
round-robin and shortest-queue-first algorithms for dynamic content
applications.
5 Fault Tolerance and Data Availability
For ease of implementation, we assume a fail-stop fault model. However,
our fault tolerance algorithm could be generalized to more complex fault
models.
At the beginning of each transaction, a scheduler
requests a sequence number from the sequencer.
Afterwards, the scheduler sends to all other schedulers
a replicate_start_transaction message containing the sequence number
for this transaction, and waits for an acknowledgment from all of them.
All other schedulers create a record with the sequence number and
the coordinating scheduler of this transaction.
No disk logging is done at this point.
If the sequencer fails, replication of the sequence
numbers on all schedulers allows for restarting the sequencer with
the last sequence number on another machine.
In case all schedulers fail, the sequence numbers
are reset everywhere (sequencer, schedulers, database proxies).
To ensure that all writes are eventually executed,
regardless of any sequence of failures in the schedulers and the
databases, each scheduler keeps a persistent log of all
write queries of committed transactions that it handled, tagged with the
transaction's sequence number.
The log is kept per table in sequence number order, to facilitate
recovery (see below).
To add data availability, the scheduler replicates this information
in the memory of all other schedulers.
Each database proxy maintains
the sequence number for the last write it committed on each table.
The database proxy does not log its state to disk.
In more detail, before a commit query is issued to any database,
the scheduler sends a replicate_end_transaction message to the other
schedulers.
This message contains the write queries that have occurred during the
transaction,
and the transaction's sequence number.
All other schedulers record the writes, augment the corresponding
remote transaction record with the commit decision, and respond
with an acknowledgment.
The originator of the replicate_end_transaction message waits for the
acknowledgments, then logs the
write queries to disk.
After the disk logging has completed,
the commit is issued to the database replicas.
For read-only transactions, the commit decision is replicated but not
logged to disk.
In the case of a single scheduler failure, all transactions of the
failed scheduler for which the live schedulers do not have a commit decision
are aborted.
A transaction for which a commit record exists, but for which a
database proxy has not yet received the commit decision is aborted at
that particular replica, and then its writes are replayed.
The latter case is, however, very rare.
In more detail, a fail-over scheduler contacts all available
database proxies.
The database proxy waits until all queued operations finish at
its database, including any pending commits.
The proxy returns to the scheduler the sequence number for the last
committed write on each database table, and the highest sequence number of any
lock request received by the database proxy.
The fail-over scheduler determines all the failed scheduler's
transactions for which a commit record exists and for which a replica
has not committed the transaction.
The reason that a replica has not committed a transaction may be
either that it did not receive the transaction's lock request or that
it did not receive the commit request.
The first case is detected by the sequence number of the transaction
being larger than the highest lock sequence number received by the proxy.
In this case, all the transaction's writes and its commit
are replayed to the proxy.
In the second case,
the fail-over scheduler first aborts the transaction and then sends the
writes
and the commit.
The database proxy also advances its value of the highest lock
sequence number received and its value of the
last sequence number of a committed write on a particular table,
as appropriate.
For all other transactions handled by the failed scheduler,
the fail-over scheduler sends an abort to all database proxies.
The database proxies rollback the specified transactions,
and also fill the gap in lock sequence numbers in case the lock
with the sequence number specified in the abort was never received.
The purpose of this, is to let active transactions of live schedulers
with higher sequence numbered locks proceed.
Each scheduler needs to keep logs for committed writes and records of
assigned lock sequence numbers until all replicas commit the
corresponding transactions.
Afterwards, these records can be garbage-collected.
When a scheduler recovers, it contacts another scheduler and replicates
its state.
In the rare case where all schedulers fail, the state is reconstructed
from the disk logs of all schedulers.
All active transactions are aborted on all database replicas,
the missing writes are applied on all databases, and all sequence
numbers are reset everywhere.
To address temporary network connection failures, each database proxy
can send a ``selective retransmission'' request for transactions it
has missed.
Specifically, the database proxy uses a timeout mechanism to detect
gaps in lock sequence that have not been filled in a given period of
time. It then contacts an available scheduler and provides its
current state.
The scheduler rolls forward the database proxy
including updating its highest lock sequence number.
When a database recovers from failure,
its database proxy contacts all available schedulers and selects one
scheduler to coordinate its recovery.
The coordinating scheduler instructs the recovering database to install
a current database snapshot from another replica, with its current
state.
Each scheduler re-establishes its connections to the database proxy and
adds the replica to its set of available machines.
The scheduler starts sending to the newly incorporated replica at
the beginning of the next transaction.
Afterwards, the database proxy becomes up-to-date by means of the
selective retransmission requests as described in the case of network
failure.
In addition, each database proxy does periodic checkpoints of its
database together with the current state (in terms of the last sequence
numbers
of its database tables).
To make a checkpoint, the database proxy stops all write operations
going out to the database engine, and when all pending write operations have
finished, it takes a snapshot of the database and writes the new state.
If any tables have not changed since the last checkpoint,
they do not need to be included in the new checkpoint.
Checkpointing is only necessary to support recovery in the unlikely case
where all database replicas fail.
In this case, each database proxy reinstalls the database from its own
checkpoint containing the
database snapshot of the last known clean state. Subsequently, recovery
proceeds as in the single database failure case above.
6 Algorithms Used for Comparison
In this section, we introduce a number of other scheduling algorithms for
comparison with conflict-aware scheduling. By gradually introducing some
of the features of conflict-aware scheduling, we are able to demonstrate
what aspects of conflict-aware scheduling contribute to its overall
performance.
Our first algorithm is an eager replication scheme that allows us
to show the benefits of asynchrony.
We then look at a number of scheduling algorithms for lazy replication.
Our second algorithm is a conventional scheduler that chooses a fixed
replica based on load at the beginning of the transaction and executes all
operations on that replica.
Our third algorithm is another fixed-replica approach, but it introduces
one feature of conflict-aware scheduling: it chooses as the fixed replica
the one that responds first to the transaction's lock request rather
than the least loaded one.
We then move away from fixed-replica algorithms, allowing different
replicas to execute reads of the same transaction, as in conflict-aware
scheduling.
Our fourth and final scheduler chooses the replica with the lowest load
at the time of the read, allowing
us to assess the difference between this approach and conflict-aware
scheduling, where a read is directed to a replica without conflicts.
We refer to these scheduler algorithms as Eager, FR-L (Fixed Replica
based on Load), FR-C (Fixed Replica based on Conflict), and VR-L (Variable
Replica based on Load). Using this terminology, the conflict-aware scheduler
would be labeled VR-C (Variable Replica based on Conflict), but we continue
to
refer to it as the conflict-aware scheduler.
In all algorithms, we use the same concurrency control mechanism,
i.e., conservative two-phase locking, the same sequence
numbering method to maintain 1-copy serializability, and the
same load balancing algorithm (see Section 4.10).
Eager follows the algorithm described by Weikum et al. [23],
which uses synchronous execution of lock requests, writes and commits on
all replicas.
In other words, the scheduler waits for completion of every
lock, write or commit operation on all replicas, before sending a
response back to the application server.
The scheduler directs a read to the replica with the lowest load.
FR-L is a conventional scheduling algorithm, in which
the scheduler is essentially a load balancer.
At the beginning of the transaction, the scheduler selects the
replica with the lowest load.
It just passes through operations
tagged with their appropriate sequence numbers.
Lock requests, writes and commits are sent to all replicas,
and the reply is sent back to the application server
when the chosen replica replies to the scheduler.
Reads are sent to the chosen replica.
The FR-L scheduler needs to record only the chosen
replica for the duration of each transaction.
6.3 Fixed Replica Based on Conflict (FR-C)
FR-C is identical to FR-L, except that the scheduler chooses
the replica that first responds to the lock request as the fixed
replica for this transaction.
As in FR-L, all reads are sent to this replica, and a response
is returned to the application server when this replica responds
to a lock request, a write or a commit.
The FR-C scheduler's state is also limited to the chosen
replica for each transaction.
In the VR-L scheduler, the response to the application server
on a lock request, write, or commit is sent as soon as the
first response is received from any replica.
A read is sent to the replica with the lowest load at the
time the read arrives at the scheduler.
This may result in the read being sent to a replica where it
needs to wait for the completion of conflicting operations
in other transactions or previous operations in its own transaction.
The VR-L scheduler needs to remember
for the duration of each replicated query
whether it has already forwarded the response and whether all machines
have responded, but, unlike a conflict-aware scheduler, it need not
remember which replicas have responded.
In other words, the size of the
state maintained is O(1) not O(N) in the number of replicas.
7 Experimental Platform
7.1 TPC-W Benchmark
The TPC-W benchmark from the Transaction Processing Council
(TPC) [22]
is a transactional web benchmark for e-commerce systems.
The benchmark simulates a bookstore.
The database contains eight tables:
customer, address, orders, order_line, credit_info, item,
author, and country.
The most frequently used are order_line, orders and credit_info, which
contain
information about the orders placed, and item and author, which
contain information about the books.
The database size is determined by
the number of items in the inventory and the size of the customer
population.
We use 100,000 items and 2.8 million customers which results in a
database of about 4 GB.
The inventory images, totaling 1.8 GB, reside on the
web server.
We implemented the fourteen different interactions specified in
the TPC-W benchmark specification. Six of the interactions are read-only,
while eight cause the database to be updated.
The read-only interactions
include access to the home page, listing of new products and
best-sellers, requests for product detail, and two interactions
involving searches.
Update transactions include user registration,
updates of the shopping cart, two order-placement transactions, and two
for administrative tasks. The frequency of execution of each
interaction is specified by the TPC-W benchmark.
The complexity of the interactions varies widely, with interactions
taking between 20 and 700 milliseconds on an unloaded machine.
The complexity of the queries varies widely as well.
In particular, the most heavyweight read queries are 50 times
more expensive than the average write query.
TPC-W uses three different workload mixes, differing in the ratio of
read-only to read-write interactions. The browsing mix contains 95%
read-only interactions, the shopping mix 80%, and the ordering mix
50%.
We implemented a client-browser emulator that allows us to vary the
load on the web site by varying the number of emulated clients.
A client session is a sequence of
interactions for the same client. For each client session, the
client emulator opens a persistent HTTP connection to the web server
and closes it at the end of the session. Each emulated client waits
for a certain think time before initiating the next interaction. The
next interaction is determined by a state transition matrix that
specifies the probability to go from one interaction to another.
The client session time and the think time are generated from a random
distribution with a mean value specified in TPC-W.
We use three popular open-source software packages: the Apache web
server [3], the PHP scripting
language [16], and the MySQL database server [14].
Since PHP is implemented as an Apache module, the web server and
application server co-exist on the same machine(s).
We use Apache v.1.3.22 for the web server,
configured with the PHP v.4.0.1 module.
We use MySQL v.4.0.1 with InnoDB transactional
extensions as our database server.
The schedulers and database proxies are both implemented with
event-driven loops that multiplex requests and responses between
the web server and the database replicas. We use FreeBSD's scalable kevent
primitive [13] to efficiently
handle thousands of connections at a single scheduler.
We use the same hardware for all machines, including those running
the client emulation software, the web servers, the schedulers and
the database engines.
Each machine has an AMD Athlon 800Mhz processor running
FreeBSD 4.1.1, 256MB
SDRAM, and a 30GB ATA-66 disk drive.
All machines are connected through a switched 100Mbps Ethernet LAN.
8 Experimental Results
The experimental results are obtained
on a cluster with 1 to 8 database server machines.
We use a number of web server machines large enough to make sure that
the
web server
stage is not the bottleneck. The largest number of web server machines
used for any experiment was 8. We use 2 schedulers to ensure data
availability.
We measure throughput in terms of the number of web interactions
per second (WIPS), the standard TPC-W performance metric.
For a given number of machines we report the peak throughput.
In other words, we vary the number of clients until we find
the peak throughput and we report the average throughput number
over several runs.
We also report average response time at this peak throughput.
Next, we break down the average query time into
query execution time, and waiting time (for locks and previous writes
in the same transaction).
Finally, we compare the performance in terms of throughput
between the conflict-aware scheduler with and without fault tolerance
and
data availability.
8.1 Throughput
Figures 3 through 5 show the throughput
of the various scheduling algorithms for each of the three
workload mixes.
In the x-axis we have the number of database machines, and
in the y-axis the number of web interactions per second.
Figure 3:
Throughput Comparison: The benefits of conflict avoidance and
fine-grained scheduling for the browsing mix.
|
Figure 4:
Throughput Comparison: The benefits of conflict avoidance and
fine-grained scheduling for the shopping mix.
|
Figure 5:
Throughput Comparison: The benefits of conflict avoidance and
fine-grained scheduling for the ordering mix.
|
First, conflict-aware scheduling outperforms all other
algorithms, and increasingly so for workload mixes with a
large fraction of writes.
Second, all asynchronous schemes outperform the eager scheme,
again increasingly so as the fraction of writes increases.
In particular, the conflict-aware protocol outperforms the eager protocol
by factors of 1.7, 2.4 and 3.5
for browsing, shopping, and ordering, respectively
at eight replicas.
Third, for the fixed replica algorithms, choosing the replica by
conflict (FR-C) rather than by load (FR-L) provides substantial
benefits, a factor of 1.4, 1.4, and 1.25 for the largest configuration,
for each of the three mixes, respectively.
Fourth, variable replica algorithms provide better results than
fixed replica algorithms, with the conflict-aware scheduler
showing a gain of a factor of 1.5, 1.6 and 2,
for browsing, shopping, and ordering, respectively,
compared to FR-L, at 8 replicas.
Finally, FR-C performs better than VR-L for the browsing and
the shopping mix, but becomes worse for the ordering mix.
Because of the larger numbers of reads in the browsing and
shopping mixes, VR-L incurs a bigger penalty for these mixes
by not sending the reads to a conflict-free replica, possibly
causing them to have to wait.
FR-C, in contrast, tries to send the reads to conflict-free replicas.
In the ordering mix, reads are fewer. Therefore, this advantage
for FR-C becomes smaller. VR-L's ability to shorten the write and
commit operations by using the first response becomes the dominant
factor.
8.2 Response Time
Figure 6 presents a comparison of the average
web interaction response time for the five scheduler algorithms and
the three workload mixes on an 8-database cluster at peak throughput.
For each workload mix, the results are normalized to the average
response time of the eager scheduler for that workload mix.
Figure 6:
Web Interaction Response Time for All Schedulers and All
Workloads, Normalized to Response Time of the Eager Scheduler
|
These results show that the conflict-aware scheduler provides better
average response times than all other schedulers.
The performance benefits of reducing conflict waiting time
are reflected in response time reductions as well, with the same
relative ranking for the different protocols as in the throughput
comparison.
8.3 Breakdown of Query Time
Figures 7, 8, and 9,
show a breakdown of the query response time into query execution
time and waiting time.
The waiting time is mostly due to conflicts; waiting for
previous writes in the same transaction is negligible.
For each workload mix,
the results are normalized to the average query response time for the
eager scheduler for that workload mix.
Figure 7:
Query Time Breakdown for All Schedulers for the Browsing
Mix, Normalized by the Query Time of the Eager Scheduler.
|
Figure 8:
Query Time Breakdown for All Schedulers for the Shopping
Mix, Normalized by the Query Time of the Eager Scheduler.
|
Figure 9:
Query Time Breakdown for All Schedulers for the Ordering
Mix, Normalized by the Query Time of the Eager Scheduler.
|
These results further stress the importance of reducing conflict
waiting time.
For all protocols, and all workloads, conflict waiting time forms
the largest fraction of the query time.
Therefore, the scheduler that reduces the conflict waiting
time the most performs the best in terms of overall throughput and
response time.
The differences in query execution time between the different
protocols are minor and do not significantly influence the overall
throughput and response time.
One might, for instance, expect the conflict-aware scheduler to produce
worse query execution times than VR-L, because of the latter's
potential for better load balancing.
VR-L has the
opportunity to direct reads to all replicas in order to balance
the load, while the conflict-aware
scheduler directs reads only to conflict-free replicas.
In practice, however, the positive effect of this extra
degree of freedom is minimal and
completely overwhelmed by the conflict-aware scheduler's reduced
conflict waiting times.
8.4 Cost of Fault Tolerance and Availability
Figure 10 shows the throughput of
conflict-aware
scheduling without fault tolerance (Unreliable), with
the overhead of logging to disk (Reliable), and with logging
to disk plus replicating the state using two and three schedulers
(Reliable-2 and Reliable-3, respectively).
All the measurements were done using the experimental
platform with 8 databases at the peak throughput.
The measurements from sections 8.1
through 8.3 correspond to the Reliable-2 bar.
Figure 10:
Overhead of Providing Fault tolerance and Various Degrees
of Availability for the Conflict-Aware Scheduler for All Workload Mixes
|
The overhead for fault tolerance and data availability
is negligible for the browsing and
shopping mixes and around 16% for the ordering mix.
This overhead is mainly due to
logging, with no extra overhead when replication of writes to remote
schedulers is added to logging (as seen by comparing the Reliable
and Reliable-2 bars).
A full checkpoint currently takes 5 minutes to perform for our 4 GB
database.
We did not include this overhead in our above measurements,
because well known techniques for minimizing
the time for taking file snapshots exist [10].
9 Simulations
We use simulation to extrapolate from our experimental results in
three different directions.
First, we explore how throughput scales if a larger cluster of
database replicas is available.
Second, we investigate the effect of faster databases, either by
using faster database software or faster machines.
Third, we show how the performance differences between the various
schedulers evolve as the conflict rate is gradually reduced.
We have developed two configurable simulators,
one for the web/application server front-ends and the other for the
database back-ends.
We use these front-end and back-end simulators
to drive actual execution of the schedulers and the database proxies.
Our motivation for running the actual scheduler code and the actual
database proxy code rather than simulating them is to
measure their overheads experimentally and determine whether or not they
may become a bottleneck for large clusters or faster databases.
The web server simulator models a very fast web server.
It accepts client requests, and sends the corresponding queries to the
scheduler without executing any application code.
The scheduler and the database proxies perform their normal functions,
but the database proxy sends each query to the database simulator
rather than to the database.
The database simulator models a cluster of database replicas.
It maintains a separate queue for each simulated replica.
Whenever a query is received from the scheduler for a particular
replica, a record is placed on that replica's queue.
The simulator estimates a completion time for the query, using the
same query execution time estimates as used for load balancing.
It polls the queues of all replicas, and sends responses when the
simulated time reaches the completion time for each query.
The simulator does not model disk I/O.
Based on profiling of actual runs, we estimate that the disk access time
is mostly overlapped with computation, due to the locality in database
accesses and the lazy database commits.
Calibration of the simulated system against measurement of the real
8-node database cluster shows that the simulated throughput
numbers are within 12% of the experimental numbers for all three mixes.
We simulate all five schedulers for all three workload mixes
for database cluster sizes up to 60 replicas.
As with the experimental results, for a given number of replicas,
we increase the number of clients until the system achieves peak
throughput,
and we report those peak throughput numbers.
The results can be found in Figures 11,
12 and 13. In the
x-axis we have the number of simulated database replicas, and in the
y-axis
the throughput in web interactions per second.
Figure 11:
Simulated Throughput Results for the Browsing Mix
|
Figure 12:
Simulated Throughput Results for the Shopping Mix
|
Figure 13:
Simulated Throughput Results for the Ordering Mix
|
The simulation results show that the experimental results obtained on
small clusters can be extrapolated to larger clusters.
In particular, the conflict-aware scheduler outperforms all other
schedulers, and the benefits of conflict awareness grow as the
cluster size grows, especially for the shopping and the ordering mix.
Furthermore, the relative order of the different schedulers remains
the same, and, in particular, all lazy schemes outperform the eager
scheduler (by up to a factor of 4.4 for the conflict-aware scheduler).
The results for the shopping mix deserve particular attention, because
they allow us to observe a flattening of the throughput of FR-C and
VR-L as the number of machines grows, a phenomenon that we could not
observe in the actual implementation.
In contrast, throughput of the conflict-aware protocol continues to
increase, albeit at a slower pace.
With increasing cluster size, the number of conflicts
increases [8].
Hence, choosing the replica based on a single criterion, either
conflict (as in FR-C) or fine-grained load balancing (as in VR-L), is
inferior to conflict-aware scheduling that combines both.
9.2.2 Bottleneck Analysis
As the cluster scales to larger numbers of machines, the following
phenomena could limit throughput increases: growth in the number
of conflicts, each replica becoming saturated with writes, or
the scheduler becoming a bottleneck.
In this section we show that the flattening
of the throughput curves for the conflict-aware scheduler in
Figures 12 and 13 is due
to conflicts among transactions, even though the scheduler seeks
to reduce conflict waiting time.
A fortiori, for the other schedulers, which invest less effort in
reducing conflicts, conflicts are even more of an impediment to
good performance at large cluster sizes.
Using the conflict-aware scheduler,
Figure 14 shows the breakdown of the
average database CPU time into idle time, time processing
reads, and time processing writes.
The breakdown is provided for each workload, for one replica and
for either the largest number of replicas simulated for that workload
or for a number of replicas at which the throughput curve has
flattened out.
Figure 14:
Breakdown of the Average Database CPU Time into Idle
time, Time for Reads, and Time for Writes, for the Browsing, Shopping and
Ordering Mixes.
|
For the browsing mix, which still scales at 60 replicas, idle time
remains low even at that cluster size.
For the shopping mix, which starts seeing some flattening out at 60
machines, idle time has grown to 15%.
For the ordering mix, which does not see any improvement in throughput
beyond 16
replicas, idle time has grown considerably, to 73%.
The fraction of write (non-idle) time
grows from under 1% for browsing and shopping and 6% for ordering on
one replica, to 8% for the browsing mix (at 60 replicas),
30% in the shopping mix (at 60 replicas), and
16% for the ordering mix (at 16 replicas).
Idle time is entirely due to conflicts. Idle time due to load imbalance
is negligible.
Most idle time occurs on a particular replica when a transaction holds
locks on the database that conflict with all lock requests in the proxy's
lock
queues, and that transaction is in the process of executing a read on a
different replica.
Additional idle time occurs while waiting for the next operation from
such a transaction.
The results in Figure 14 clearly show
that idle time, and thus conflicts, is the primary impediment to
scaling.
Write saturation (a replica being fully occupied with writes) does not
occur.
For the sizes of clusters simulated, the scheduler is not a bottleneck.
While the scheduler CPU usage grows linearly with
increases in cluster size, one scheduler is enough
to sustain the maximum configuration for all three mixes.
Table 1 shows the CPU, memory, disk
and network usage at the scheduler for the shopping mix, in a
configuration with one scheduler and 60 replicas.
The CPU usage reaches about 58%, while all other resource usage is very
low.
For all other mixes, the resource usage is lower at their corresponding
maximum configuration.
Table 1:
Resource Usage at the Scheduler for the Shopping Mix at 60 Replicas
CPU |
Memory |
Network |
Disk |
(%) |
(MB) |
(MB/sec) |
(MB/sec) |
58% |
6.3 |
3.8 |
0.021 |
|
If the database is significantly faster, either by using more powerful
hardware or by using a high-performance database engine, a
conflict-aware scheduler continues to provide good throughput scaling.
In Figure 15 we show throughput as a function of
the number of replicas for databases twice and four times faster than
the MySQL database we use in the experiments.
This is the highest speed of database for which we could simulate a
cluster with 60 replicas.
We simulate faster databases by reducing the estimated length of each
query in the simulation.
Figure 15 shows that the faster databases produce
similar scaling curves with correspondingly higher throughputs.
Three schedulers are necessary in the largest configuration with the
fastest database.
Figure 15:
Simulated Throughput Results with Faster Database Replicas
for the Shopping Mix
|
Table-level conservative two-phase locking, as used in our
implementation, causes a high conflict rate.
We investigate the benefits of conflict-aware scheduling under conflict
rates as low as 1% of that observed in the experimental workload.
Figures 16 and 17
compare throughput as a function of cluster size
between the conflict-aware scheduler on one hand, and the eager and
the conventional lazy FR-L schedulers on the other hand.
We vary the conflict rate from 1% to 100% of the conflict
rate observed in the experimental workload.
In particular, if a table-level conflict occurs, we ignore the
conflict with the specified probability.
Figure 16:
Simulated Throughput Results for Various Conflict Rates
for the Conflict-Aware and the Eager Scheduler for the Shopping Mix
|
Figure 17:
Simulated Throughput Results for Various Conflict Rates
for the Conflict-Aware and the FR-L Scheduler for the Shopping Mix
|
Obviously, the performance differences become smaller as the number
of conflicts decreases, but at a 1% conflict rate and at the maximum
cluster size,
the conflict-aware protocol is still a factor
of 1.8 better than Eager, and a factor of 1.3 better than a lazy
protocol without any optimizations (FR-L).
This result demonstrates that conflict-awareness continues to offer
benefits for workloads with lower conflict rates or systems with finer-grain
concurrency control.
10 Related Work
Current high-volume web servers such as the official web server used for
the Olympic games [6] and real-life e-commerce sites based on
IBM's WebSphere Commerce Edition [11],
rely on expensive supercomputers to satisfy the
volume of requests. Nevertheless, performance of such servers may
become a problem during periods of peak load.
Neptune [18] adopts a primary-copy approach to providing
consistency in a partitioned service cluster.
Their scalability study is limited to web applications with loose
consistency requirements, such as bulletin boards and auction sites, for
which
scaling is easier to achieve.
They do not address e-commerce workloads or other web applications
with stronger consistency requirements.
Zhang et al. [25] have
previously attempted to scale dynamic content
sites by using clusters in their HACC project.
They extend a technique from clustered static-content servers,
locality-aware request distribution [15], to work in dynamic
content sites.
Their study, however, is limited to read-only workloads.
In a more general dynamic content server, replication implies the need
for consistency maintenance.
Replication has previously been
used mainly for fault tolerance and data availability [7].
Gray et al. [8] shows that classic solutions based
on eager replication which provide serializability
do not scale well.
Lazy replication algorithms with asynchronous update
propagation used in wide-area applications [17,21] scale
well, but also expose inconsistencies to the user.
More recently, asynchronous replication based on group
communication [12,20,24]
has been proposed
to provide serializability and scaling at the same time.
This approach is implemented inside the database layer.
Each replica functions independently.
A transaction acquires locks locally.
Prior to commit, the database sends the other
replicas the write-sets.
Conflicts are solved by each replica independently.
This implies the need to abort transactions when a write-set
received from a remote transaction conflicts with a local transaction.
This approach differs from ours in that we consider a cluster in which
a scheduler can direct operations to certain replicas, while they
consider a more conventional distributed database setting in which
a transaction normally executes locally.
Also, our approach is implemented outside of the database.
TP-monitors, such as Tuxedo [9], are superficially similar
in functionality to our scheduler.
They differ in that they provide programming support for
replicated application servers and for accessing different databases using
conventional two-phase commit, not transparent support for
replicating a database for throughput scaling.
11 Conclusions
We have described conflict-aware scheduling, a lazy replication
technique for a cluster of database replicas serving as a back-end
to a dynamic content site.
A conflict-aware scheduler enforces 1-copy serializability by
assigning transaction sequence numbers, and it reduces conflict
waiting time by directing reads to replicas where no conflicts exist.
This design matches well the characteristics of
the database workloads that we have observed in dynamic content sites,
namely high locality, high cost of reads relative to the cost of
writes, and high conflict rates.
No modifications are necessary in the application server or in the
database to take advantage of a conflict-aware scheduler.
We have evaluated conflict-aware scheduling, both by measurement
of an implementation and by simulation.
We use software platforms in common use: the Apache web server,
the PHP scripting language, and the MySQL database.
We use the various workload mixes of the TPC-W benchmark
to evaluate overall scaling behavior and the contribution
of our scheduling algorithms.
In an 8-node cluster, the conflict-aware scheduler brings factors
of 1.5, 1.6 and 2 in throughput improvement,
compared to a conflict-oblivious lazy scheduler,
and factors of 1.7, 2.4 and 3.5, compared to an eager scheduler,
for the browsing, shopping and ordering mixes, respectively.
Furthermore, our simulations show that conflict-aware schedulers
scale well to larger clusters and faster machines, and that they
maintain an edge over eager and conflict-oblivious schedulers even
if the conflict rate is much lower.
Acknowledgments
We would like to thank Gustavo Alonso and Andre Schiper for their
advice on early drafts of this paper.
We also thank our shepherd, Mike Dahlin, for his guidance,
and the anonymous reviewers for their comments.
The work also benefited from informal discussions with
Emmanuel Cecchet and Karthick Rajamani, and from the
contributions of the DynaServer team towards a
better understanding of dynamic content benchmarks.
- 1
-
C. Amza, E. Cecchet, A. Chanda, A. Cox, S. Elnikety, R. Gil, J. Marguerite,
K. Rajamani, and W. Zwaenepoel.
Specification and implementation of dynamic web site benchmarks.
In 5th IEEE Workshop on Workload Characterization, November
2002.
- 2
-
C. Amza, A. Cox, and W. Zwaenepoel.
Scaling and availability for dynamic content web sites.
Technical Report TR02-395, Rice University, 2002.
- 3
-
Apache Software Foundation.
https://www.apache.org/.
- 4
-
P.A. Bernstein, V. Hadzilacos, and N. Goodman.
Concurrency Control and Recovery in Database Systems.
Addison-Wesley, 1987.
- 5
-
H. Boral, W. Alexander, L. Clay, G. Copeland, S. Danforth, M. Franklin,
B. Hart, M. Smith, and P. Valduriez.
Prototyping Bubba, A Highly Parallel Database System.
In IEEE Transactions on Knowledge and Data Engineering,
volume 2, pages 4-24, March 1990.
- 6
-
J. Challenger, A. Iyengar, K. Witting, C. Ferstat, and P. Reed.
A Publishing System for Efficiently Creating Dynamic Web Data.
In Proceedings of IEEE INFOCOM 2000, March 2000.
- 7
-
R. Flannery.
The Informix Handbook.
Prentice Hall, 2000.
- 8
-
J. Gray, P. Helland, P. O'Neil, and D. Shasha.
The dangers of replication and a solution.
In Proceedings of the 1996 ACM SIGMOD, June, pages
173-182, 1996.
- 9
-
J. Gray and A. Reuter.
Transaction Processing : Concepts and Techniques.
1993.
- 10
-
N. Hutchinson, S. Manley, M. Federwisch, G. Harris, D. Hitz, S. Kleiman, and
S. O'Malley.
Logical vs. physical file system backup.
In Proceedings of the Third USENIX Symposium on Operating System
Design and Implementation, February 1999.
- 11
-
A. Jhingran.
Anatomy of a real e-commerce system.
In Proceedings of the Association for Computing Machinery
Special Interest Group on Management of Data, May 2000.
- 12
-
B. Kemme and G. Alonso.
Don't be lazy, be consistent: Postgres-R, a new way to implement
Database Replication.
In Proceedings of the 26th International Conference on Very
Large Databases, September 2000.
- 13
-
J. Lemon.
Kqueue: A generic and scalable event notification facility.
In Proceedings of the 2001 Annual Usenix Technical Conference,
June 2001.
- 14
-
MySQL.
https://www.mysql.com.
- 15
-
V. S. Pai, M. Aron, G. Banga, M. Svendsen, P. Druschel, W. Zwaenepoel, and
E. Nahum.
Locality-aware request distribution in cluster-based network servers.
In Proceedings of the Eighth International Conference on
Architectural Support for Programming Languages and Operating Systems, pages
205-216, October 1998.
- 16
-
PHP Hypertext Preprocessor.
https://www.php.net.
- 17
-
Y. Saito and H. Levy.
Optimistic Replication for Internet Data Services.
In International Symposium on Distributed Computing, October
2000.
- 18
-
K. Shen, T. Yang, L. Chu, J. L. Holliday, D. Kuschner, and H. Zhu.
Neptune: Scalable Replica Management and Programming Support for
Cluster-based Network Services.
In Proceedings of the Third USENIX Symposium on Internet
Technologies and Systems, pages 207-216, March 2001.
- 19
-
Slashdot: Handling the Loads on 9/11.
https://slashdot.org.
- 20
-
I. Stanoi, D. Agrawal, and A. El Abbadi.
Using broadcast primitives in replicated databases.
In Proceedings of the IEEE International Conference
on Distributed Computing Systems ICDCS'98, pages 148-155, May 1998.
- 21
-
D. B. Terry, M. M. Theimer, K. Petersen, A. J. Demers, M. J. Spreitzer, and
C. H. Hauser.
Managing update conflicts in bayou, a weakly connected replicated
storage system.
In Proceedings 15th Symposium on Operating Systems Principles,
December 1995.
- 22
-
Transaction Processing Council.
https://www.tpc.org/.
- 23
-
G. Weikum and G. Vossen.
Transactional Information Systems. Theory, Algorithms and the
Practice of Concurrency Control and Recovery.
Addison-Wesley, Reading, Massachusetts, second edition, 2002.
- 24
-
M. Wiesmann, F. Pedone, A. Schiper, B. Kemme, and G. Alonso.
Database replication techniques: a three parameter classification.
In Proceedings of the 19th IEEE Symposium on Reliable
Distributed Systems (SRDS2000), October 2000.
- 25
-
X. Zhang, M. Barrientos, J. B. Chen, and M. Seltzer.
HACC: An architecture for cluster-based web servers.
In Proceedings of the 2000 Annual Usenix Technical Conference,
June 2000.
Cristiana Amza (amza@cs.rice.edu)
|